Molecular Modeling & Data Science at J-STAR Research and Porton USA
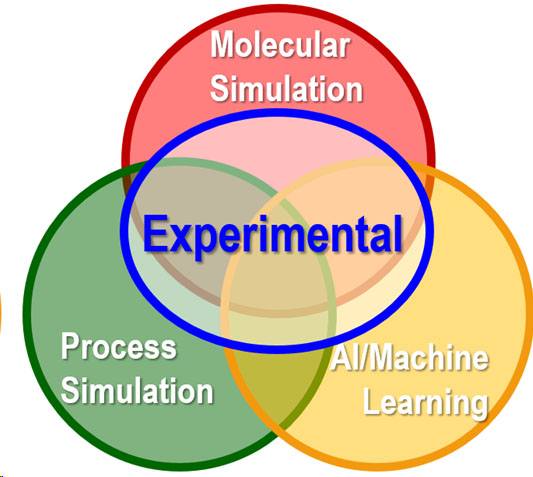
With advances in computational materials science and process simulation, computational applications are becoming integral part of the drug development and manufacturing workflow.[1][2] These computational methods facilitate easier navigation through the complex multidimensional space of pharmaceutical process development tasks. Therefore, by combining computational and experimental approaches, pharmaceutical development can be de-risked, resulting in significant time and cost savings.
The breadth and diversity of pharmaceutical development tasks dictates the wide range of the computational capabilities and application adopted for projects support at J-Star Research/Porton
Computational Capabilities
We utilize a wide range of computational methods—from molecular mechanics to quantum chemistry and data science—to study systems that vary from small molecules to new modalities in gas, liquid, and solid phases. Selection of the method depends on a compromise between the expected accuracy of predictions and the computational feasibility of the application; and the available amount of historical data for potential machine learning (ML) model building.
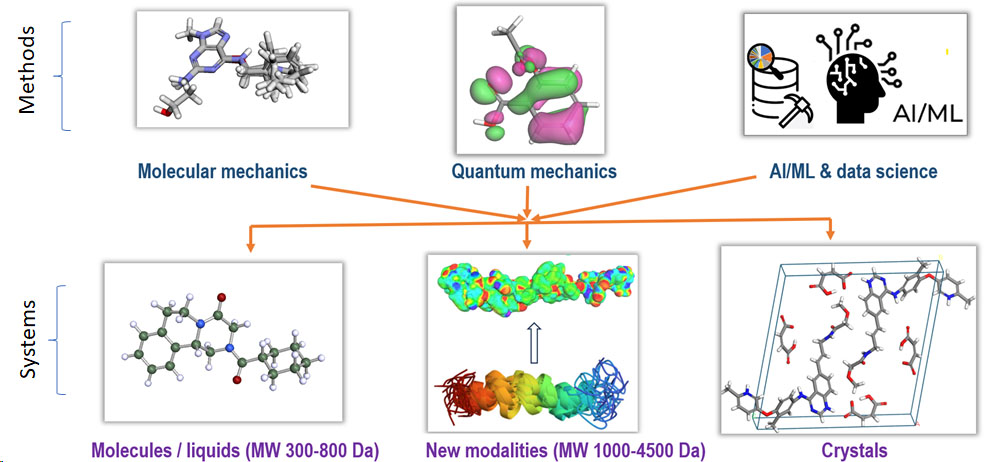
Applications
Molecular simulation and data science approaches for combined computational and experimental project support could be divided into three categories: virtual screening approaches, properties characterization and optimization, and AI/ML-based DoE calculations.
All applications were validated on multiple internal and external projects, and average performance evaluation provided for some of the approaches below.
Computational Applications
Virtual solvent screening for pharmaceutical crystallization | Virtual coformer/counterion screening for cocrystal/salt crystallization | Virtual solvent and solid form screening for impurity(ies) rejection |
Virtual excipients screening for coprocessing | Observed crystal form characterization along salt-cocrystal spectrum | Crystal shape optimization |
Mechanical properties prediction | Molecular and crystal form analytical properties prediction | Chemometrics analysis of analytical spectra |
AI/ML Based DoE |
Virtual Screening for Crystallization, Form Screening and Coprocessing
Virtual screening is a computational technique used to rank a database of compounds (for example, solvents, coformers or counterions) to identify the most promising solutions for a specific task. [1][3][4] A subset of the most favorable compounds is recommended for a targeted experimental follow up.
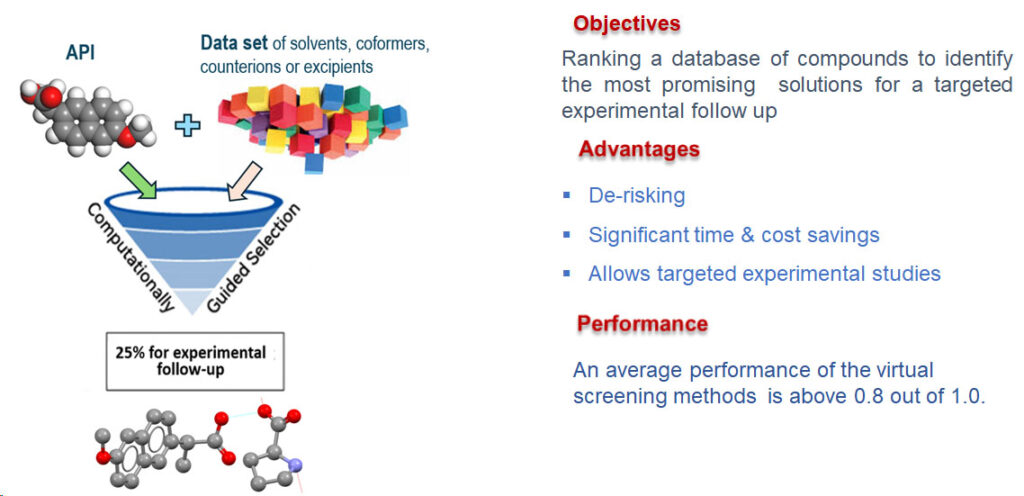
Virtual Solvent and Solid Form Screening for Impurity(ies) Rejection
Rejection of impurities forming a solid solution with an API or intermediate in crystalline phase is typically the most challenging task to achieve, particularly if a single (re)crystallization is desired. To enable efficient impurity rejection, the most comprehensive model was recently developed and validated, which considers the following contributions to impurities uptake/rejection by crystallization.[5]
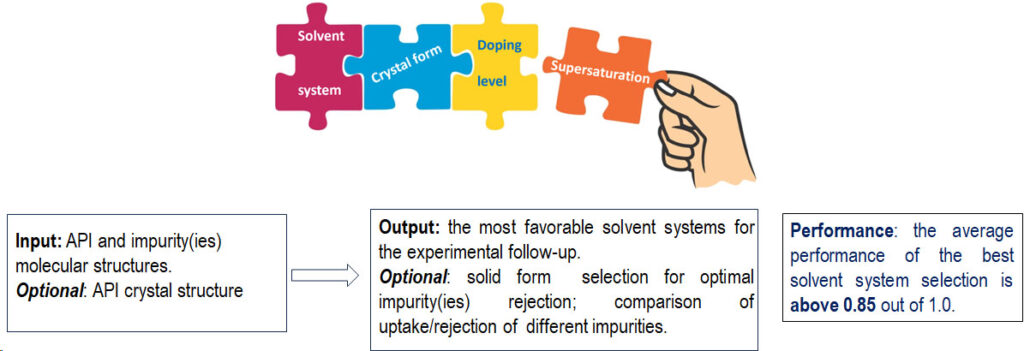
Molecular and Solid Form Characterization & Optimization
- Observed crystal form characterization along salt-cocrystal spectrum by DMol3 or CASTEP.
- Analytical properties prediction: (ss)NMR, IR, Raman, Uv-vis by quantum chemistry tools Gaussian16, DMol3 , or CASTEP.
- Solubility and solubility improvement prediction by COSMOtherm or DynoChem.
- Crystal shape prediction and optimization by solvent virtual screening using ADDICT, or Materials Studio and COSMOtherm.
- Mechanical properties prediction by CASTEP or DMol3.
- Prediction of relative miscibility and solubility of APIs in polymers

Observed Crystal Form Characterization along Salt-Cocrystal Spectrum
Definitive characterization of pharmaceutical salt and cocrystal solid forms is crucial from regulatory and intellectual property perspectives. However, due to the low X-ray scattering power, caution should be exercised when determining proton locations in pharmaceutical acid-base systems based solely on single-crystal X-ray diffraction refinement. High accuracy and reliability of computational approaches for describing cocrystal and salt solid forms have been demonstrated and utilized to projects support.[6]
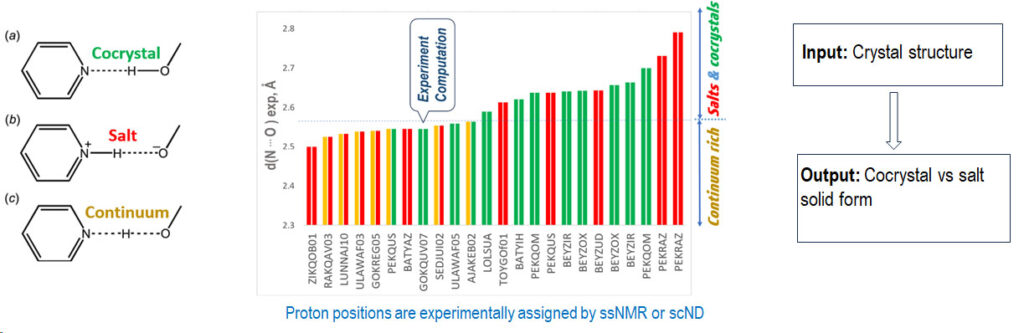
Chemometrics Analysis of Spectral Data
Chemometric analysis of spectral data enables the extraction of meaningful information from complex spectroscopic datasets, such as those obtained from infrared or Raman spectrometers. This analysis helps in examining the chemical composition of samples by identifying patterns and relationships within the spectral data. Common computational techniques include clustering, principal component analysis (PCA), and the development of classification or regression machine learning (ML) models based on spectral data.
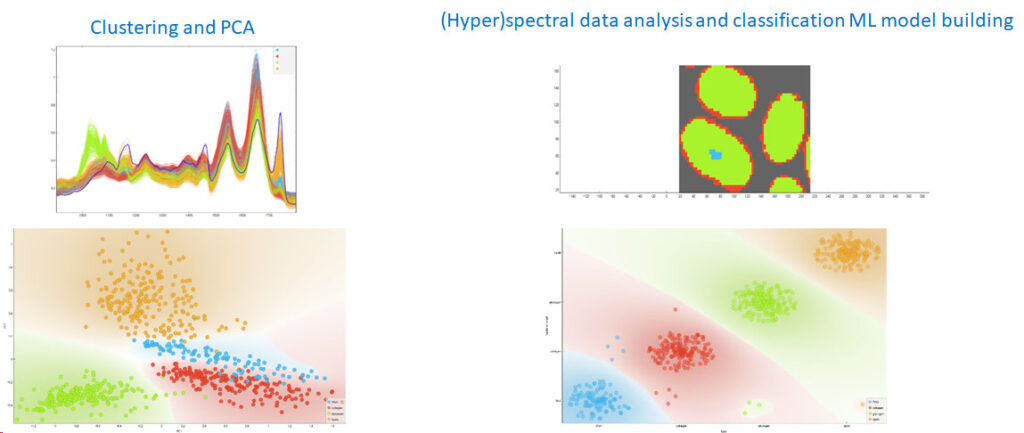
AI/ML-based DoE for process and method optimization
We apply SuntheticsML AI/ML-DoE platform to reduce the numbers of experiments required to optimize multidimensional process development for crystallization, process chemistry, analytical chemistry, etc. The method allows for fast and reliable convergence to the optimal parameter space for target properties using Bayesian optimization approach.
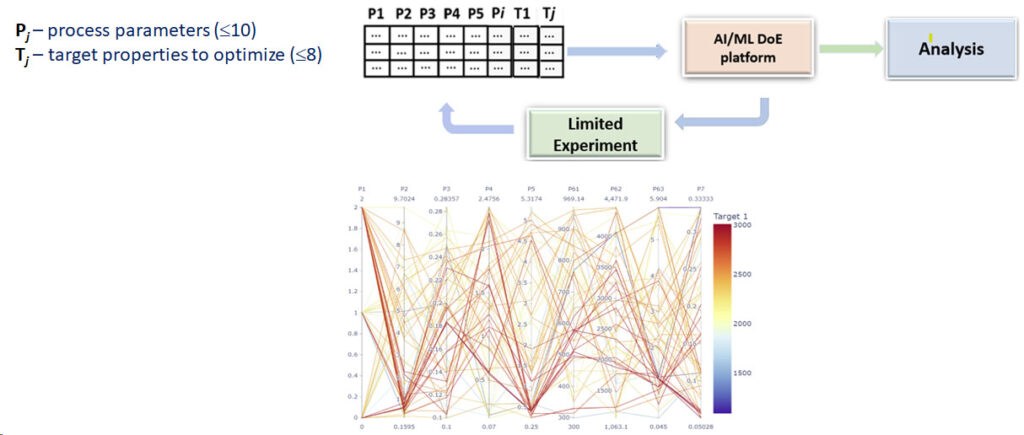
References
1. Abramov, Y. A.; Sun, G.; Zeng, Q. J. Chem. Inf. Model. 2022, 62, 1160.
2. Abramov, Y.A., Ed. Computational Pharmaceutical Solid State Chemistry, John Wiley & Sons, 2016.
3. Abramov, Y.A.; Loschen, C.; Klamt, A. J. Pharm. Sci., 2012, 101, 3687.
4. Shah, H.S.; Michelle, C.; Xie, T.; Chaturvedi, K.; Kuang. S.; Abramov, Y.A. Pharm Res. 2023, 40, 2779.
5. Abramov, Y.A.; Zelellow, A.; Chen, C.-Y.; Wang,J.; Sekharan, S. Cryst. Growth Des. 2022, 12, 6844.
6. Abramov, Y.A.; Wang, J. Cryst. Growth Des. 2024, 24, 4017.